
We introduce deformable convolution to our architecture, which has proven its effectiveness on object detection and semantic segmentation, with a strong ability to adapt to different geometric structures. Furthermore, to avoid manually choosing the most appropriate receptive field size of each stage, Second, based on our discovery of different receptive field requirements of the two stages, we design different architectures for them. Our model still has two stages, but in contrast to the direct concatenation of two separate networks, we propose a set of techniques to strengthen the entanglement of the two stages, such as shuffling features from the former model to the latter one and using intermediate supervision to reinforce the learning of each stage. In this paper, we propose an end-to-end architecture specifically designed for the SRD task.
#Super denoising for windows 1080p
5.4 shows that we can reduce the time-consuming of a 8spp rendering to almost one-fifth via reducing the resolution from 1080p to 540p. We call this new task SRD (Super-Resolution and Denoising) and focus on providing such a neural network in this paper. The heavy burden of producing a high quality result is then shifted onto the following neural network, which needs to excel in both super-resolution and denoising while maintaining high computational efficiency. The advantage of this approach is obvious: by reducing both resolution and sampling rate, the computational cost of the main bottleneck of the pipeline: Monte Carlo rendering, is greatly reduced. This leads to a low-resolution, noisy initial result, which will then be processed by a novel neural network to perform joint denoising and super-resolution. In this work, we aim to further improve the efficiency of path tracing by investigating a new strategy that has not been explored before: reducing the resolution and the sampling rate at the same time. In contrast, sample-based denoising methods (Gharbi et al., 2019 Munkberg and Hasselgren, 2020) work in the sample space, filtering individual raw samples at each pixel location.
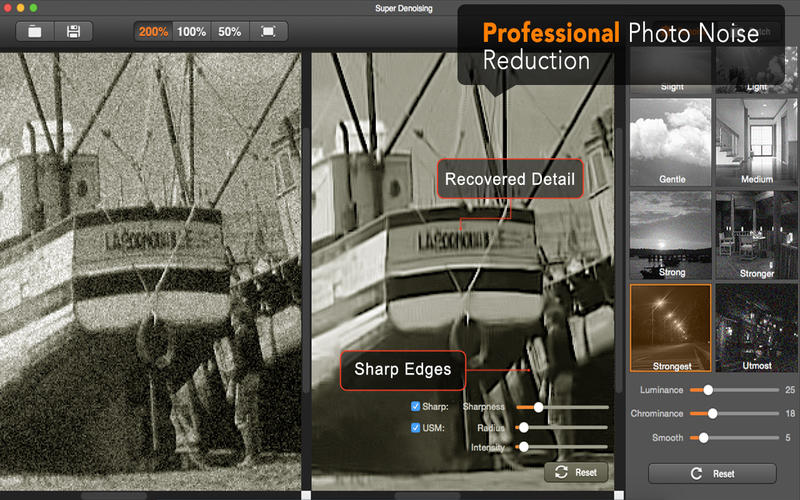
Specifically, pixel-based denoising methods (Chaitanya et al., 2017 Bako et al., 2017 Vogels et al., 2018 Xu et al., 2019) either directly regress the final pixel colors or predict pixel-centric filters. DNNs are then used to denoise the output to restore clean imagery. The latter renders imagery at the desired resolution directly but with fewer color samples per pixel, leading to noisy output. (Xiao et al., 2020) to increase its resolution while maintaining image sharpness. The former uses path tracing to render lower resolution imagery, then applies upsampling techniques such as DLSS (Edelsten et al., 2019), or temporal neural network by Lei et al. Previous methods in this domain adopt two different methodologies: super-resolution and denoising. Following these successes some have also tried to use neural networks to accelerate path-tracing-based rendering. Recently, deep neural networks (DNNs) have shown great potential in many visual rendering tasks either for speeding up the pipeline, or improving the visual quality of the rendered results. Previous methods and their variants adopted for the SRD task. Extensive experiments show that the proposed method outperforms That leads to the introduction of deformable convolution into the networkĭesign. We discover that denoisingĪnd super-resolution require very different receptive fields, a key insight ItĬontains two cascaded stages with shared components. The main contribution of this work is a newĮnd-to-end network architecture, specifically designed for the SRD task. Tracing, which is then fed into a deep neural network to produce a Low-resolution and fewer-sample (thus noisy) image to be rendered by path
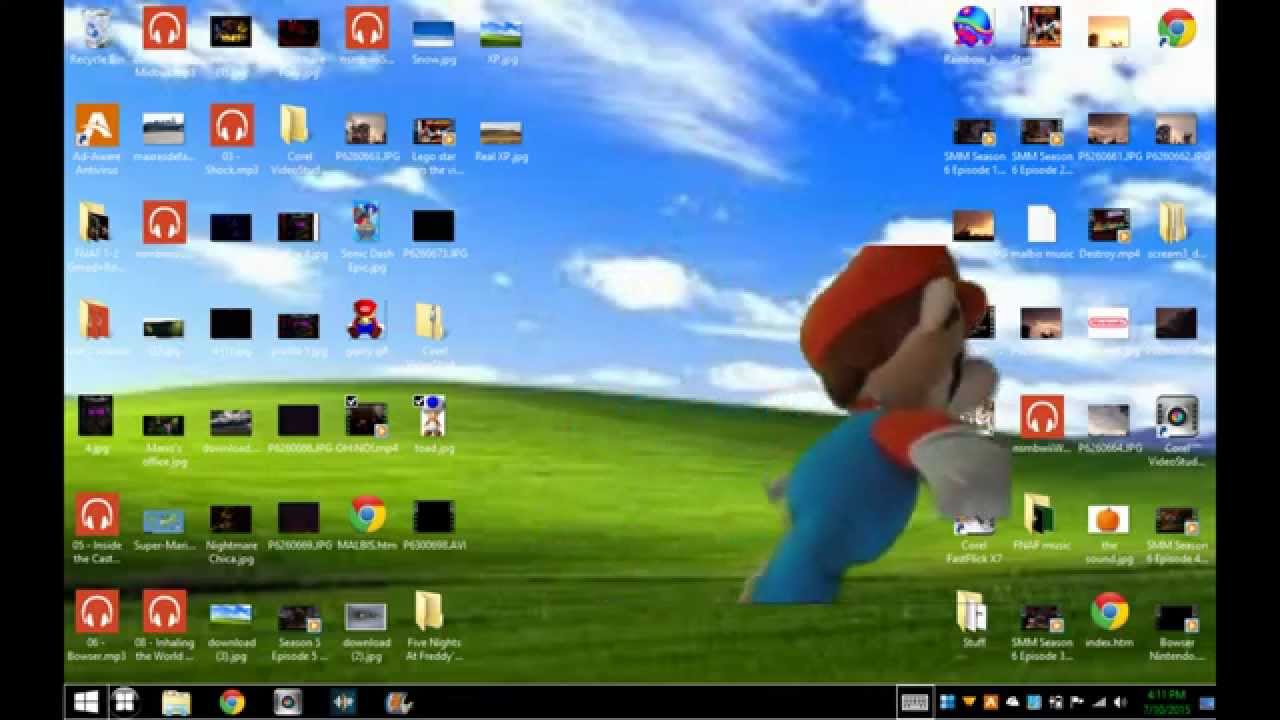
This new type of joint filtering allows only a Path tracing can be further accelerated by joint super-resolution and denoising However, denoising and super-resolution have only beenĬonsidered separately in previous work. Rendering with super-resolution or denoising neural networks in Recent works make use of deep neural networks toĪccelerate this process, by improving either low-resolution or fewer-sample The classic Monte Carlo path tracing can achieve high quality rendering at
